技术路线 |
描述 |
总时间 |
加速比 |
备注 |
Baseline |
串行程序 |
207 s |
1 |
|
more3omp |
0.4+5+0.3 |
23.0s |
|
|
时间细划,初始化omp |
0.03+5+0.1 |
21.2s |
|
|
不换算法,必须加锁 |
特别满 |
|
|
|
扫描行算法 |
0.03+2.2+0.1 |
18.5s |
|
|
扫描行算法 + task动态线程池 |
|
26s |
|
|
扫描行算法 + task动态线程池 + 延迟发射 |
|
26s |
|
|
扫描行算法 + task动态线程池 + 延迟发射 |
|
26s |
|
|
扫描行算法 + 化解重复,提高粒度:每个线程一行,不同线程杜绝同一行扫描行算法 |
但是没并行起来 |
106s |
|
|
扫描行算法 + 常驻64线程 |
|
86s |
|
|
## 初始时间 |
|
|
|
|
Time taken is 21.364595 6.066717 EnforceLabelConnectivityComputing
|
|
|
|
|
## 时间细划,初始化omp |
|
|
|
|
细致划分,malloc size大小的空间不耗时,是初始化为-1耗时 |
|
|
|
|
Time taken is 16.963094 0.000025 EnforceLabelConnectivity numlable
Time taken is 17.395982 0.432887 EnforceLabelConnectivity xvec yvec
Time taken is 22.668060 5.272079 EnforceLabelConnectivity iteration
Time taken is 23.001499 0.333438 EnforceLabelConnectivity klabelsComputing
|
|
|
|
|
修改后 |
|
|
|
|
Time taken is 16.063057 0.000026 EnforceLabelConnectivity numlable
Time taken is 16.095485 0.032428 EnforceLabelConnectivity xvec yvec
Time taken is 21.116599 5.021114 EnforceLabelConnectivity iteration
Time taken is 21.237874 0.121275 EnforceLabelConnectivity klabelsComputing
|
|
|
|
|
## 改 dx4,dy4 实现访存连续性 |
|
|
|
|
但是可能会导致adjlabel的值不对,导致结果不对 |
|
|
|
|
4分钟+, 满核结束不了,已经混乱了。
5分钟+,满核结束不了,大翻车。
可能的原因:
1. 本来不是计算密集型,加锁导致是串行,而且还有sz次锁的获取与释放的开销。
2. 某个线程改了nlabels,其余运行时读取可能还要同步修改。
我又想到是不是只有一个锁,有没有多个锁的实现。还是超时结束不了。
omp_set_lock(&lock[nindex]); //获得互斥器
if( 0 > nlabels[nindex] && labels[oindex] == labels[nindex] )
{
xvec[count] = x;
yvec[count] = y;
nlabels[nindex] = label;
count++;
}
omp_unset_lock(&lock[nindex]); //释放互斥器
多个锁满足了nlabels的竞争,但是count的竞争还是只能一个锁。除非将数组保存变成队列才有可能,因为没计数器了。
好耶,segmentation fault (core dumped)。果然读到外面去了。
不好耶了,并行的地方加了锁,还是会
double free or corruption (out) //内存越界之类的
200~400行不等seg fault。
然后我打了时间戳
可以看出至少前面是正常的。
多运行几次,有时候segfault,有时corruption,我服了。
但是位置好像还是在上面的循环
每次报错位置还不一样,但是迭代的点还是对的。
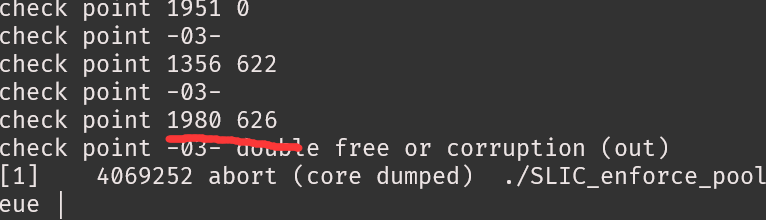
https://stackoverflow.com/questions/32227321/atomic-operation-on-queuet
munmap_chunk(): invalid pointer
黑人问号?纳尼
没办法,只能加锁,读取,写入都加锁,但是就是特别慢,4分钟+。
omp_set_lock(&lock); //获得互斥器
qindex = workq.front();
workq.pop();
omp_unset_lock(&lock); //释放互斥器
omp_set_lock(&lock2); //获得互斥器
if( 0 > nlabels[nindex] && labels[oindex] == labels[nindex] )
{
nlabels[nindex] = label;
workq2.push(nindex);
saveq.push(nindex);
}
omp_unset_lock(&lock2); //释放互斥器
读取,写入不是同一个队列,尝试用2个锁,还是特别慢,5分钟根本跑不完。
q.front()变成了q.top()
扫描线算法至少比每像素算法快一个数量级。
Time taken is 16.144375 13.062605 PerformSuperpixelSegmentation_VariableSandM 循环
Time taken is 16.144399 0.000025 EnforceLabelConnectivity numlable
Time taken is 16.177300 0.032901 EnforceLabelConnectivity xvec yvec
Time taken is 48.978709 32.801409 EnforceLabelConnectivity iteration
Time taken is 49.086252 0.107543 EnforceLabelConnectivity klabelsComputing time=49086 ms
There are 86475718 points' labels are different from original file.
不知道哪里错了,需要debug。简单debug,发现小问题。
Time taken is 15.670141 0.000024 EnforceLabelConnectivity numlable
Time taken is 15.718014 0.047873 EnforceLabelConnectivity xvec yvec
Time taken is 22.103680 6.385666 EnforceLabelConnectivity iteration
Time taken is 22.219160 0.115480 EnforceLabelConnectivity klabelsComputing time=22219 ms
There are 0 points' labels are different from original file.
但是尴尬的是并没有快。哭哭哭
~~。
优化一下变量,快了3秒,大胜利!!!
Time taken is 16.203514 0.000029 EnforceLabelConnectivity numlable
Time taken is 16.234977 0.031463 EnforceLabelConnectivity xvec yvec
Time taken is 18.428990 2.194013 EnforceLabelConnectivity iteration
Time taken is 18.527664 0.098674 EnforceLabelConnectivity klabelsComputing time=18527 ms
There are 0 points' labels are different from original file.
虽然我在总结里写了,很难控制。但是,哎,我就是不信邪,就是玩😉
喜提segfault,打印task调用,发现task从上到下,之字形调用,而且没用一个结束的。按照设想,横向x增加比调用task快的,现在好像task堵塞的样子。
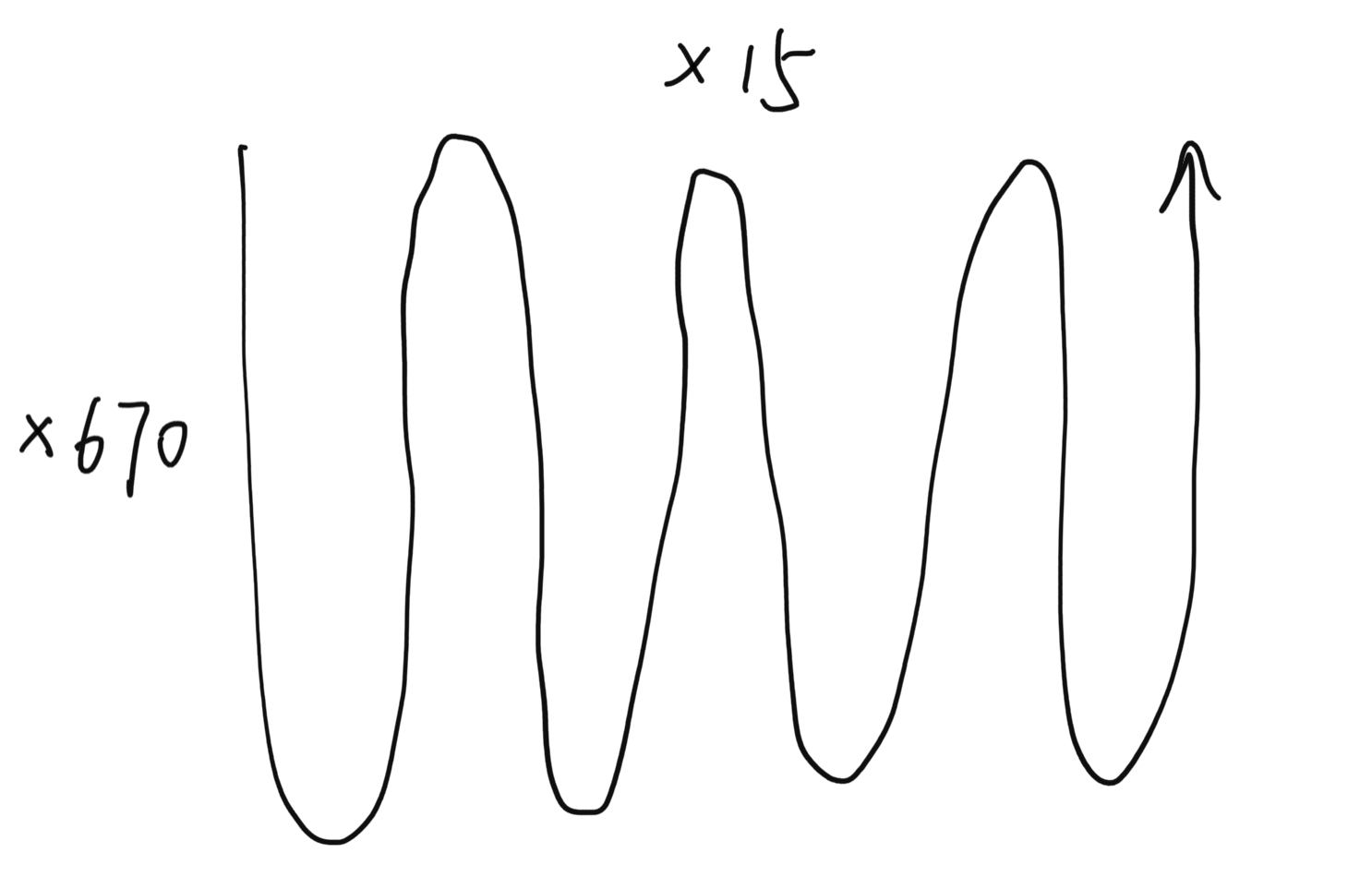
好像是没加,但是结果不对
#pragma omp parallel num_threads(64)
{
#pragma omp single
让我们仔细分析一下是怎么偏离预期的:
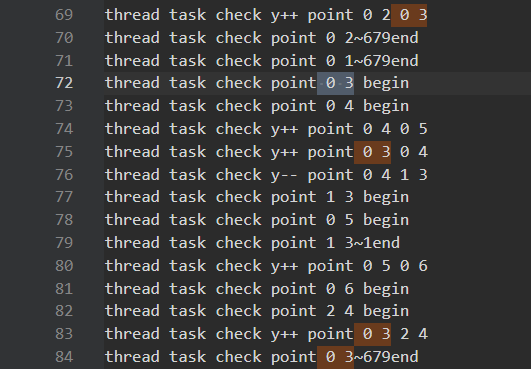
1. (0,2)调用(0,3),(0,3)调用(0,4)很正常。但是(0,3)竟然调用了(2,4),这说明(0,3)循环到(1,3)时,发现(1,4)是已经处理的,而(2,4)是未处理的。进一步说明了(0,4)在被(0,3)创建了之后,先一步循环到(1,4),并将其处理。

2. (0,4)先循环到(1,4),反手还调用(1,3)。然后由于(0,3)调用了(2,4)。导致(0,4)循环到后面以为到(2,4)就截止了。
3. 虽然我说不出有什么问题,但是这不受控制的混乱调用,肯定不是我们想见的。
尝试把占用时间的print去掉。时间不短(重复调用),还是错的。(后面才发现,错误是threadcount,threadq里,每次循环完忘记清空了。日~)
Time taken is 16.226124 0.000024 EnforceLabelConnectivity numlable
Time taken is 16.258697 0.032573 EnforceLabelConnectivity xvec yvec
Time taken is 26.320222 10.061525 EnforceLabelConnectivity iteration
Time taken is 26.401399 0.081177 EnforceLabelConnectivity klabelsComputing time=26401 ms
There are 86588716 points' labels are different from original file.
Time taken is 15.743455 0.000025 EnforceLabelConnectivity numlable
Time taken is 15.773654 0.030198 EnforceLabelConnectivity xvec yvec
Time taken is 26.348979 10.575326 EnforceLabelConnectivity iteration
Time taken is 26.442129 0.093150 EnforceLabelConnectivity klabelsComputing time=26442 ms
There are 0 points' labels are different from original file.
现在的想法是要有先后顺序,把对(x,y)一行都处理完,再发射task。或者采取延迟发射的。
- 把发射任务(x+delay,y)用队列存储,每次循环check一下,最后循环结束后,在全部发射。
- 或者标记(x+delay,y)发射(x,y)。但是对于循环结束后的,不好处理。
Time taken is 17.344073 0.000027 EnforceLabelConnectivity numlable
Time taken is 17.377535 0.033462 EnforceLabelConnectivity xvec yvec
Time taken is 28.461901 11.084366 EnforceLabelConnectivity iteration
Time taken is 28.544698 0.082797 EnforceLabelConnectivity klabelsComputing time=28544 ms
There are 86588716 points' labels are different from original file.
很奇怪,结果不对。难道是delay的值太小。
把delay的值从10调整到750,甚至是2600,大于宽度了,结果还是不对。这是不对劲的,因为这时相当于把对(x,y)一行都处理完,再发射task。
这时我才感觉到是其他地方写错了,错误是threadcount,threadq里,每次循环完忘记清空了。日~
delay = 2600 结果是对了,但是也太慢了,至少要比串行快啊?
Time taken is 15.538704 0.000026 EnforceLabelConnectivity numlable
Time taken is 15.577671 0.038968 EnforceLabelConnectivity xvec yvec
Time taken is 28.233859 12.656188 EnforceLabelConnectivity iteration
Time taken is 28.332256 0.098396 EnforceLabelConnectivity klabelsComputing time=28332 ms
delay = 20 快了一点,哭
Time taken is 15.631368 0.000025 EnforceLabelConnectivity numlable
Time taken is 15.661496 0.030128 EnforceLabelConnectivity xvec yvec
Time taken is 26.788105 11.126609 EnforceLabelConnectivity iteration
Time taken is 26.869487 0.081382 EnforceLabelConnectivity klabelsComputing time=26869 ms
There are 0 points' labels are different from original file.
-
打上时间戳
end Time 84 32839 taken is 0.000000 dxy4
end Time 84 32839 taken is 0.000000 threadcount
end Time 84 32839 taken is 0.031285 core
end Time 84 32839 taken is 0.000023 count
说明还是并行没写好。
-
检查是否调用64核,htop显示是64核
- 猜测原因
- 产生了大量重复的任务,还是划分的原因,上下限制了之后,左右的重复情况如何化解。
- 每个进程一行,task分配到y%64号线程去。但是openmp的task好像不能指定线程号。
- 任务压入第y%64个队列,线程从队列取任务。
- eg,第3行的后面两个线程,threadcount=0,无作用。
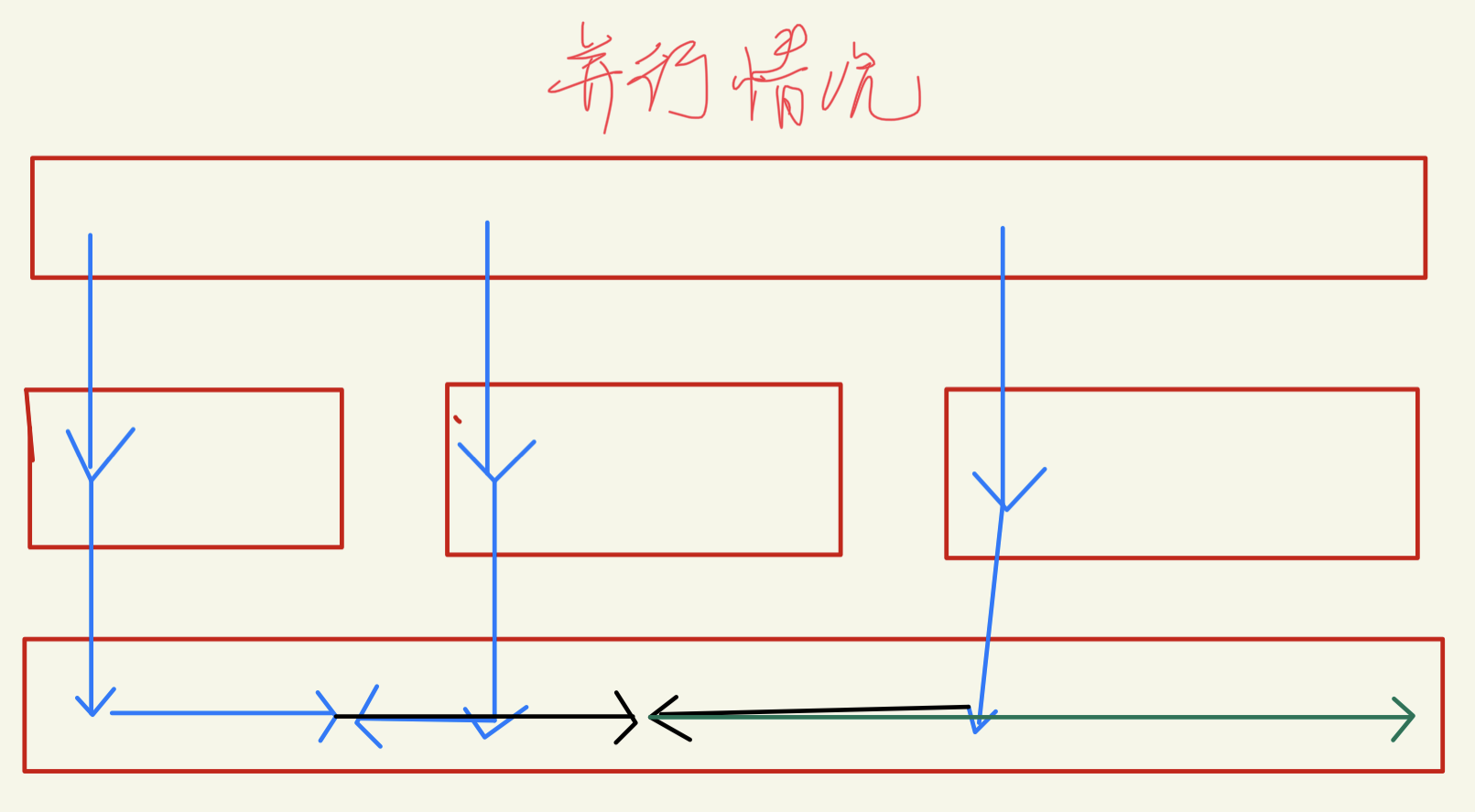
- 有许多任务量过小的情况,粒度不够,次数还多,导致调用产生的开销大
- task的线程池就是不靠谱
- 可以行分割或列分割,根据输入
- 任务压入第y%64个队列,线程从队列取任务。
- 但是这里同一队列的写入与读取又冲突了。可以用64个双队列,一写一读。在交换的时候等待+同步。
- 不同线程写入同一个也冲突,每个线程再来64个队列保存,同步的时候再汇总写入。
想法很美好,但是最后的效果并不是每次64线程,基本都只有1-5个任务。导致近似单线程还有调用开销。(node6有人,node5慢些)
Time taken is 36.212269 32.876626 PerformSuperpixelSegmentation_VariableSandM 循环
Time taken is 36.212297 0.000028 EnforceLabelConnectivity numlable
Time taken is 36.247536 0.035239 EnforceLabelConnectivity xvec yvec
Time taken is 106.097341 69.849805 EnforceLabelConnectivity iteration
Time taken is 106.204154 0.106813 EnforceLabelConnectivity klabelsComputing time=106204 ms
There are 0 points' labels are different from original file.
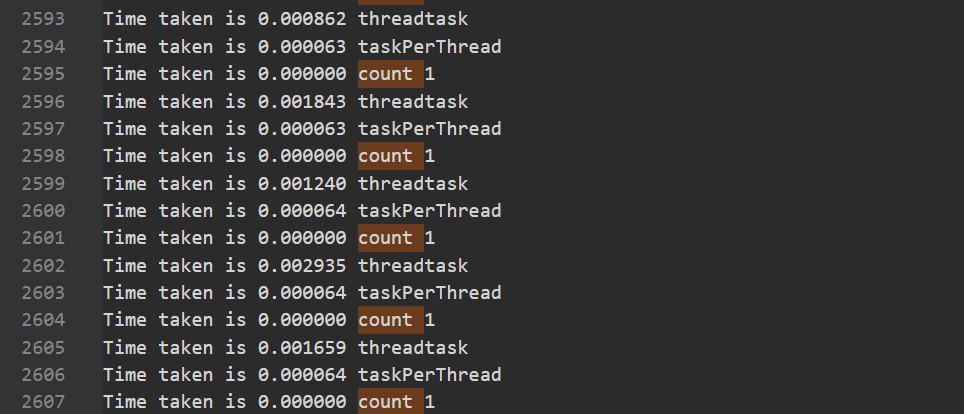
这个原因感觉是一开始只有1个,然后一般也就产生1/2个任务。将其初始任务改成64个就行。
但是如何一开始启动64个呢,我又提前不知道任务。
写完又是segFault,debug
1. [64][64][10000]太大了,每次的队列应该没这么多[64][64][100]
2. 对于结束的统计,要用同步一下,需要加critical。结果就对了
但是,这也太慢了
Time taken is 28.219408 0.000017 EnforceLabelConnectivity numlable
Time taken is 28.271994 0.052586 EnforceLabelConnectivity xvec yvec
Time taken is 83.591540 55.319546 EnforceLabelConnectivity iteration
Time taken is 83.696990 0.105450 EnforceLabelConnectivity klabelsComputing time=83696 ms
There are 0 points' labels are different from original file.
没时间研究
没时间研究
感觉要自己写个结构体
1. 数据可以无序
2. 最好数据各异
3. 支持并行读每个元素(数组?
4. 支持并行写一堆元素,并且维护size大小
暂无
在这次并行中,让我意识到几点
1. 任务的划分一定不能重复,相互干扰。比如,四邻域泛洪任务重复会导致竞争问题,需要加锁。但是,描绘线,任务不重复,直接避免了加锁的低效。而且重复会导致计算重复,同时占用线程。
2. 并行任务的结果,如果不是一定要存在同一个变量就分开存,既不需要线程私有变量,最后归约;也不会存同一个位置导致竞争。比如,这次的任务会产生一堆不相关的index,那直接每个线程一个数组存,既不会冲突,之后还能接着并行。或者用更大的sz大小数组存index,结果更不会冲突了。
3. 对于任务数增加且不确定的情况,不推荐使用task进程。因为自动调度很难控制,既不知道迭代了多少,也不确定之后会不会有隐藏的竞争。 推荐类似双队列的调度,确定一批任务,并行一批任务,同步一批任务的结果,然后重新并行。
1. 问题:中间并行一批任务的时候还是记得分开存结果。同步的时候再处理一下就行。
2. 双队列可能有任务量过少的问题,导致变单线程。
3. 想到了一种启动64常驻线程,产生任务又等待任务的结构。但是问题是:任务的保存要满足产生任务的写入和处理任务的读取。在不考虑写爆的情况(循环),维护数组的写入与读取位置是可行的。任务的结束通过每个线程在读取不到任务时,判断自己发布的所有任务也被完成了,标记自己发布的任务完成了。所有发布的任务都完成,再结束。
好吧,我感觉我分析了一堆,就是在放屁。还是串行快,这个问题就难划分。就不是并行的算法。
这次编程遇到的问题,大多数如下:
- 对每次循环开始时所以变量的清空,重新赋初值
- 结束时,全部清空。
无