PyG是一个基于PyTorch的用于处理不规则数据(比如图)的库,或者说是一个用于在图等数据上快速实现表征学习的框架。它的运行速度很快,训练模型速度可以达到DGL(Deep Graph Library )v0.2 的40倍(数据来自论文)。除了出色的运行速度外,PyG中也集成了很多论文中提出的方法(GCN,SGC,GAT,SAGE等等)和常用数据集。因此对于复现论文来说也是相当方便。
经典的库才有函数可以支持,自己的模型,自己根据自动微分实现。还要自己写GPU并行。
MessagePassing 是网络交互的核心
torch_geometric.data.Data (下面简称Data) 用于构建图
- 每个节点的特征 x
- 形状是[num_nodes, num_node_features]。
- 节点之间的边 edge_index
- 形状是 [2, num_edges]
- 节点的标签 y
- 假如有。形状是[num_nodes, *]
- 边的特征 edge_attr
- [num_edges, num_edge_features]
通过data.face来扩展Data
在 PyG 中,我们使用的不是这种写法,而是在get()函数中根据 index 返回torch_geometric.data.Data类型的数据,在Data里包含了数据和 label。
由于是无向图,因此有 4 条边:(0 -> 1), (1 -> 0), (1 -> 2), (2 -> 1)。每个节点都有自己的特征。上面这个图可以使用 torch_geometric.data.Data
来表示如下:
import torch
from torch_geometric.data import Data
# 由于是无向图,因此有 4 条边:(0 -> 1), (1 -> 0), (1 -> 2), (2 -> 1)
edge_index = torch.tensor([[0, 1, 1, 2],
[1, 0, 2, 1]], dtype=torch.long)
# 节点的特征
x = torch.tensor([[-1], [0], [1]], dtype=torch.float)
data = Data(x=x, edge_index=edge_index)
注意edge_index中边的存储方式,有两个list,第 1 个list是边的起始点,第 2 个list是边的目标节点。注意与下面的存储方式的区别。
import torch
from torch_geometric.data import Data
edge_index = torch.tensor([[0, 1],
[1, 0],
[1, 2],
[2, 1]], dtype=torch.long)
x = torch.tensor([[-1], [0], [1]], dtype=torch.float)
data = Data(x=x, edge_index=edge_index.t().contiguous())
这种情况edge_index需要先转置然后使用contiguous()方法。关于contiguous()函数的作用,查看 PyTorch中的contiguous。
import torch
from torch_geometric.data import InMemoryDataset
class MyOwnDataset(InMemoryDataset): # or (Dataset)
def __init__(self, root, transform=None, pre_transform=None):
super(MyOwnDataset, self).__init__(root, transform, pre_transform)
self.data, self.slices = torch.load(self.processed_paths[0])
# 返回一个包含没有处理的数据的名字的list。如果你只有一个文件,那么它返回的list将只包含一个元素。事实上,你可以返回一个空list,然后确定你的文件在后面的函数process()中。
@property
def raw_file_names(self):
return ['some_file_1', 'some_file_2', ...]
# 很像上一个函数,它返回一个包含所有处理过的数据的list。在调用process()这个函数后,通常返回的list只有一个元素,它只保存已经处理过的数据的名字。
@property
def processed_file_names(self):
return ['data.pt']
def download(self):
pass
# Download to `self.raw_dir`. or just pass
# 整合你的数据成一个包含data的list。然后调用 self.collate()去计算将用DataLodadr的片段。
def process(self):
# Read data into huge `Data` list.
data_list = [...]
if self.pre_filter is not None:
data_list [data for data in data_list if self.pre_filter(data)]
if self.pre_transform is not None:
data_list = [self.pre_transform(data) for data in data_list]
data, slices = self.collate(data_list)
torch.save((data, slices), self.processed_paths[0])
DataLoader 这个类允许你通过batch的方式feed数据。创建一个DotaLoader实例,可以简单的指定数据集和你期望的batch size。
loader = DataLoader(dataset, batch_size=512, shuffle=True)
DataLoader的每一次迭代都会产生一个Batch对象。它非常像Data对象。但是带有一个‘batch’属性。它指明了了对应图上的节点连接关系。因为DataLoader聚合来自不同图的的batch的x,y 和edge_index,所以GNN模型需要batch信息去知道那个节点属于哪一图。
for batch in loader:
batch
>>> Batch(x=[1024, 21], edge_index=[2, 1568], y=[512], batch=[1024])
其中,x 表示表格节点的 embedding,e 表示边的特征,ϕ 表示 message 函数,□ 表示聚合 aggregation 函数,γ 表示 update 函数。上标表示层的 index,比如说,当 k = 1 时,x 则表示所有输入网络的图结构的数据。
为了实现这个,我们需要定义:
- message
- 定义了对于每个节点对 (xi,xj),怎样生成信息(message)。
- update
- aggregation scheme
- propagate(edge_index, size=None, **kwargs)
- 这个函数最终会按序调用 message、aggregate 和 update 函数。
- update(aggr_out, **kwargs)
- 这个函数利用聚合好的信息(message)更新每个节点的 embedding。
- edge_index (Tensor or SparseTensor)
- 输入的边的信息,定义底层图形连接/消息传递流。
- torch.LongTensor类型
- its shape must be defined as
[2, num_messages]
, where messages from nodes in edge_index[0]
are sent to nodes in edge_index[1]
- torch_sparse.SparseTensor类型
- its sparse indices (row, col) should relate to row = edge_index[1] and col = edge_index[0].
- 也不一定是方形节点矩阵。x=(x_N, x_M).
会根据 flow=“source_to_target”和if flow=“target_to_source”或者x_i,x_j,来区分处理的边。
x_j表示提升张量,它包含每个边的源节点特征,即每个节点的邻居。通过在变量名后添加_i或_j,可以自动提升节点特征。事实上,任何张量都可以通过这种方式转换,只要它们包含源节点或目标节点特征。
_j表示每条边的起点,_i表示每条边的终点。x_j表示的就是每条边起点的x值(也就是Feature)。如果你手动加了别的内容,那么它的_j, _i也会自动进行处理,这个自己稍微单步执行一下就知道了
在实现message的时候,节点特征会自动map到各自的source and target nodes。
aggregation scheme 只需要设置参数就好,“add”, “mean”, “min”, “max” and “mul” operations
aggregation 输出作为第一个参数,后面的参数是 propagate()的
\[
\mathbf{x}_i^{(k)} = \sum_{j \in \mathcal{N}(i) \cup \{ i \}} \frac{1}{\sqrt{\deg(i)} \cdot \sqrt{\deg(j)}} \cdot \left( \mathbf{\Theta}^{\top} \cdot \mathbf{x}_j^{(k-1)} \right)
\]
该式子先将周围的节点与权重矩阵\theta相乘, 然后通过节点的度degree正则化,最后相加
步骤可以拆分如下
- 添加self-loop 到邻接矩阵(Adjacency Matrix)。
- 节点特征的线性变换。
- 计算归一化系数
- Normalize 节点特征。
- sum相邻节点的feature(“add”聚合)。
步骤1 和 2 需要在message passing 前被计算好。 3 - 5 可以torch_geometric.nn.MessagePassing 类。
添加self-loop的目的是让featrue在聚合的过程中加入当前节点自己的feature,没有self-loop聚合的就只有邻居节点的信息。
import torch
from torch_geometric.nn import MessagePassing
from torch_geometric.utils import add_self_loops, degree
class GCNConv(MessagePassing):
def __init__(self, in_channels, out_channels):
super().__init__(aggr='add') # "Add" aggregation (Step 5).
self.lin = torch.nn.Linear(in_channels, out_channels)
def forward(self, x, edge_index):
# x has shape [N, in_channels]
# edge_index has shape [2, E]
# Step 1: Add self-loops to the adjacency matrix.
edge_index, _ = add_self_loops(edge_index, num_nodes=x.size(0))
# Step 2: Linearly transform node feature matrix.
x = self.lin(x)
# Step 3: Compute normalization.
row, col = edge_index
deg = degree(col, x.size(0), dtype=x.dtype)
deg_inv_sqrt = deg.pow(-0.5)
deg_inv_sqrt[deg_inv_sqrt == float('inf')] = 0
norm = deg_inv_sqrt[row] * deg_inv_sqrt[col]
# Step 4-5: Start propagating messages.
return self.propagate(edge_index, x=x, norm=norm)
def message(self, x_j, norm):
# x_j has shape [E, out_channels]
# Step 4: Normalize node features.
return norm.view(-1, 1) * x_j
所有的逻辑代码都在forward()里面,当我们调用propagate()函数之后,它将会在内部调用message()和update()。
conv = GCNConv(16, 32)
x = conv(x, edge_index)
聚合函数(aggregation)我们用最大池化(max pooling),这样上述公示中的 AGGREGATE 可以写为:
上述公式中,对于每个邻居节点,都和一个 weighted matrix 相乘,并且加上一个 bias,传给一个激活函数。相关代码如下(对应第二个图):
class SAGEConv(MessagePassing):
def __init__(self, in_channels, out_channels):
super(SAGEConv, self).__init__(aggr='max')
self.lin = torch.nn.Linear(in_channels, out_channels)
self.act = torch.nn.ReLU()
def message(self, x_j):
# x_j has shape [E, in_channels]
x_j = self.lin(x_j)
x_j = self.act(x_j)
return x_j
对于 update 方法,我们需要聚合更新每个节点的 embedding,然后加上权重矩阵和偏置(对应第一个图第二行):
class SAGEConv(MessagePassing):
def __init__(self, in_channels, out_channels):
self.update_lin = torch.nn.Linear(in_channels + out_channels, in_channels, bias=False)
self.update_act = torch.nn.ReLU()
def update(self, aggr_out, x):
# aggr_out has shape [N, out_channels]
new_embedding = torch.cat([aggr_out, x], dim=1)
new_embedding = self.update_lin(new_embedding)
new_embedding = torch.update_act(new_embedding)
return new_embedding
综上所述,SageConv 层的定于方法如下:
import torch
from torch.nn import Sequential as Seq, Linear, ReLU
from torch_geometric.nn import MessagePassing
from torch_geometric.utils import remove_self_loops, add_self_loops
class SAGEConv(MessagePassing):
def __init__(self, in_channels, out_channels):
super(SAGEConv, self).__init__(aggr='max') # "Max" aggregation.
self.lin = torch.nn.Linear(in_channels, out_channels)
self.act = torch.nn.ReLU()
self.update_lin = torch.nn.Linear(in_channels + out_channels, in_channels, bias=False)
self.update_act = torch.nn.ReLU()
def forward(self, x, edge_index):
# x has shape [N, in_channels]
# edge_index has shape [2, E]
# Removes every self-loop in the graph given by edge_index, so that (i,i)∉E for every i ∈ V.
edge_index, _ = remove_self_loops(edge_index)
# Adds a self-loop (i,i)∈ E to every node i ∈ V in the graph given by edge_index
edge_index, _ = add_self_loops(edge_index, num_nodes=x.size(0))
return self.propagate(edge_index, size=(x.size(0), x.size(0)), x=x)
def message(self, x_j):
# x_j has shape [E, in_channels]
x_j = self.lin(x_j)
x_j = self.act(x_j)
return x_j
def update(self, aggr_out, x):
# aggr_out has shape [N, out_channels]
new_embedding = torch.cat([aggr_out, x], dim=1)
new_embedding = self.update_lin(new_embedding)
new_embedding = self.update_act(new_embedding)
return new_embedding
GNN的batch实现和传统的有区别。
将网络复制batch次,batchSize的数据产生batchSize个Loss。通过Sum或者Max处理Loss,整体同时更新所有的网络参数。至于网络中循环输入和输出的H(t-1)和Ht。(感觉直接平均就行了。
有几个可能的问题
1. 网络中参数不是线性层,CNN这种的网络。pytorch会自动并行吗?还需要手动
2. 还有个问题,如果你还想用PyG的X和edge。并不能额外拓展维度。
通过 rescaling or padding(填充) 将相同大小的网络复制,来实现新添加维度。而新添加维度的大小就是batch_size。
但是由于图神经网络的特殊性:边和节点的表示。传统的方法要么不可行,要么会有数据的重复表示产生的大量内存消耗。
为此引入了ADVANCED MINI-BATCHING来实现对大量数据的并行。
https://pytorch-geometric.readthedocs.io/en/latest/notes/batching.html
- 邻接矩阵以对角线的方式堆叠(创建包含多个孤立子图的巨大图)
- 节点和目标特征只是在节点维度中串联???
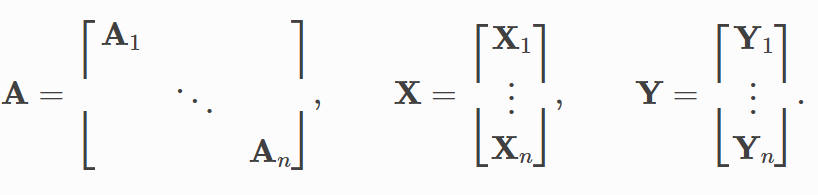
- 依赖message passing 方案的GNN operators不需要修改,因为消息仍然不能在属于不同图的两个节点之间交换。
- 没有计算或内存开销。例如,此batching 过程完全可以在不填充节点或边特征的情况下工作。请注意,邻接矩阵没有额外的内存开销,因为它们以稀疏方式保存,只保存非零项,即边。
可以实现将多个图batch成一个大图。 通过重写collate()来实现,并继承了pytorch的所有参数,比如num_workers.
在合并的时候,除开edge_index [2, num_edges]通过增加第二维度。其余(节点)都是增加第一维度的个数。
# 原本是[2*4]
# 自己实现的话,是直接连接
>>> tensor([[0, 0, 1, 1, 0, 0, 1, 1],
[0, 1, 1, 2, 0, 1, 1, 2]])
# 会修改成新的边
print(batch.edge_index)
>>> tensor([[0, 0, 1, 1, 2, 2, 3, 3],
[0, 1, 1, 2, 3, 4, 4, 5]])
from torch_geometric.data import Data
from torch_geometric.loader import DataLoader
data_list = [Data(...), ..., Data(...)]
loader = DataLoader(data_list, batch_size=32)
from torch_geometric.datasets import TUDataset
from torch_geometric.loader import DataLoader
dataset = TUDataset(root='/tmp/ENZYMES', name='ENZYMES', use_node_attr=True)
loader = DataLoader(dataset, batch_size=32, shuffle=True)
for batch in loader:
batch
>>> DataBatch(batch=[1082], edge_index=[2, 4066], x=[1082, 21], y=[32])
batch.num_graphs
>>> 32
暂无
暂无
无